Contrasting carefully Textual dialogue is found in most books and movies, the language of everyday interaction tends to be chaotic and patchy, full of false starts, interruptions, and people talking to each other.
From casual conversations between friends to squabbles between siblings to formal discussions in the boardroom, real conversation anarchism. It seems that anyone can learn a miracle language Absolutely, given the random nature of language experience.
For this reason, many linguists – including Noam Chomsky, founder of modern linguistics – believed that language learners need some kind of glue to curb the unusual nature of everyday language. This glue is grammar: a system of rules for generating grammatical sentences.
Children must have a Template rules wired into their brains To help them overcome the limitations of their language experience – or so the thinking goes.
This form might, for example, contain an “upper rule” that specifies how new pieces are added to existing statements. Children then only need to see if their native language is the same, such as English, where the verb goes before the object (eg “I am eating” sushi”), or one like Japanese, where the verb goes after the object (in Japanese, the same sentence is structured as “I eat sushi”).
But new insights into language learning come from an unexpected source: artificial intelligence. A new breed of large AI language models Newspaper articles can be writtenAnd the PoetryAnd the computer code And the Answer the questions honestly After being exposed to huge amounts of language input. And most surprisingly, they all do this without the help of rules.
language without grammar
Even if their choice of words is sometimes strange, illogical, or contains Racism, sexism and other harmful prejudices, one thing is very clear: the vast majority of the output of these AI language models is grammatically correct. However, there are no grammatical templates or grammar installed in it – it relies on linguistic experience alone, however messy it may be.
GPT-3, arguably the most famous of these models, is a giant deep learning neural network that contains 175 billion variables. He was trained to predict the next word in a sentence by looking at what came before across hundreds of billions of words from the Internet, books, and Wikipedia. When he made the wrong prediction, his parameters were modified using automatic learning algorithm.
Significantly, GPT-3 can generate plausible text that reacts to prompts such as “The last movie summary” Fast and the Furious “is…” or “Write a poem in Emily Dickinson style”. Furthermore it, GPT-3 can respond To SAT level analogies, reading comprehension questions and even simple arithmetic problems – all from learning how to predict the next word.
Comparing AI models and human brains
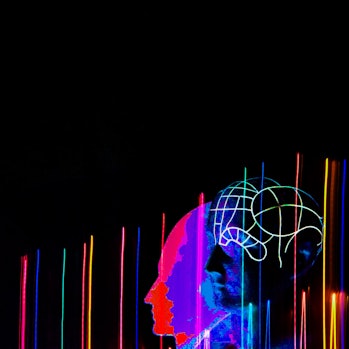
But the similarity with human language does not stop there. Posted in natural neuroscience Show that these artificial deep learning networks appear to be using The same mathematical principles as the human brain.
Led search group Neuroscientist Uri Hassonfirst compare how good GPT-2 – GPT-3’s “little brother” – Humans can predict the next word in a story taken from the “This American Life” podcast: People and AI predicted the exact same word nearly 50 percent of the time.
The researchers recorded the volunteers’ brain activity while they listened to the story. The best explanation for the activation patterns they observed was that people’s brains – such as GPT-2 – were not just using one or two previous words when making predictions but relied on the accumulated context of up to 100 previous words.
Altogether, the authors conclude: “Our finding of spontaneous predictive neural signals while participants listened to natural speech suggests that active prediction may underlie human lifelong learning of language.”
One potential concern is that these new language models of AI are fed a lot of input: GPT-3 has been trained on language experience equivalent to 20,000 human years. But Preliminary study which has yet to be peer-reviewed found that GPT-2 can still model human next-word predictions and activate the brain even when trained on just 100 million words. This is well within the amount of language input an average child would do You hear during the first ten years of life.
We do not suggest that GPT-3 or GPT-2 learn language just like children. In fact, these AI models don’t seem to absorb much, if any, of what they’re saying, while understanding is fundamental to human use of language. However, what these models prove is that a learner – albeit a silicon – can learn a language well enough from just exposure to produce perfectly fine grammatical sentences and do so in a way similar to the processing of the human brain.
Rethink language learning
For years, many linguists believed that language learning was impossible without a built-in grammar template. New AI models provide otherwise. They demonstrate that the ability to produce grammatical language can be learned from linguistic experience alone. Likewise, we suggest that Kids don’t need innate rules to learn the language.
The old saying goes “Children should be seen, not listened to,” but the latest models of AI language suggest that nothing could be further from the truth. Instead, children should be too Take part in the conversation back and forth As much as possible to help them develop their language skills. Language expertise – not grammar – is the key to becoming a competent user of language.
This article was originally published Conversation by Morten H. Christiansen and Pablo Contreras Callins at Cornell University. Read the The original article is here.